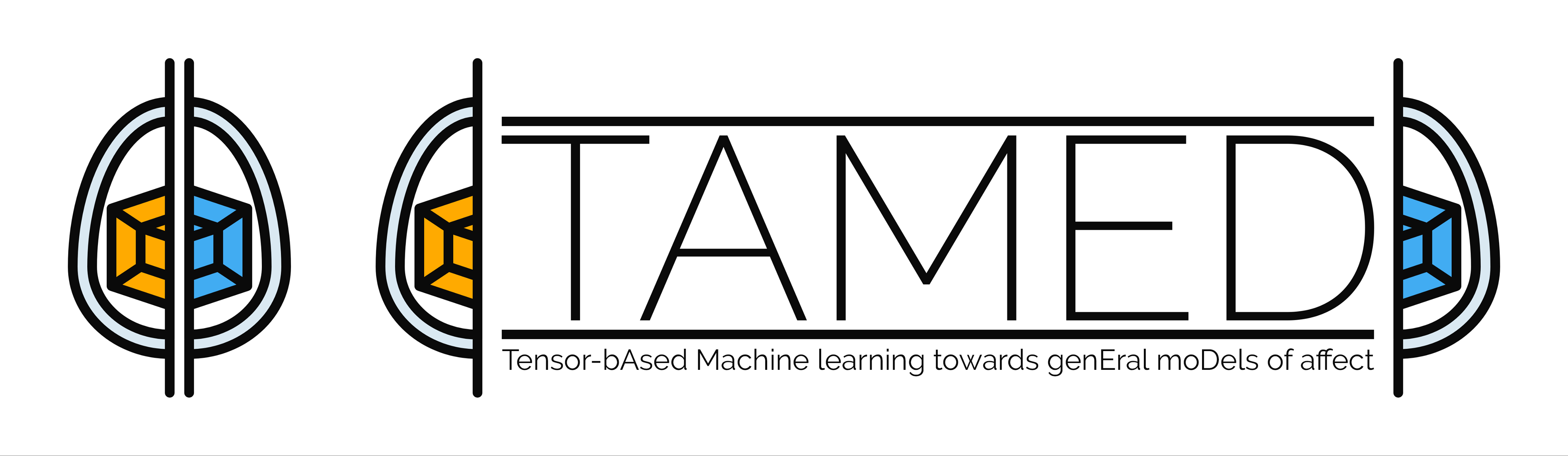
The pixels and sounds of emotion! What if we could detect emotions in a general, user-agnostic fashion? Is it possible to capture human emotion solely by looking at the pixels of the screen and hearing the sounds of the interaction? No sensors, no access to biometrics, facial expressions or speech!
We are thrilled to present our new IEEE Transactions on Affective Computing paper by Kostas Makantasis, Georgios Yannakakis, and Antonios Liapis in which we transfer and introduce the idea of general-purpose deep representations for gameplaying to affective computing. We show we can predict arousal via audiovisual game footage across 4 very different games with top accuracies (as high as 85%) using the demanding leave-one-video-out validation scheme.
What if emotion could be captured in a general and subject-agnostic fashion? Is it possible, for instance, to design general-purpose representations that detect affect solely from the pixels and audio of a human-computer interaction video? In this paper we address the above questions by evaluating the capacity of deep learned representations to predict affect by relying only on audiovisual information of videos. We assume that the pixels and audio of an interactive session embed the necessary information required to detect affect. We test our hypothesis in the domain of digital games and evaluate the degree to which deep classifiers and deep preference learning algorithms can learn to predict the arousal of players based only on the video footage of their gameplay. Our results from four dissimilar games suggest that general-purpose representations can be built across games as the arousal models obtain average accuracies as high as 85% using the challenging leave-one-video-out cross-validation scheme. The dissimilar audiovisual characteristics of the tested games showcase the strengths and limitations of the proposed method.
Fast-paced arcade games featuring a top-down camera perspective, clear forms and colours to distinguish game objects, and loud sound effects tied to game events, help the model predict affect accurately only from audiovisual information.
The work is supported by the H2020 Marie Curie Widening Fellowship TAMED Tensor-based ML towards General Models of Affect and AI4Media projects.
Come and study with us! M.Sc. and PhD.
Or sign up to keep up to date with our research and activities!